“From a Whisper in my head to a Symphony in my hands: Proposing a Combinatorial Therapy Design in Acute Myeloid Leukaemia”
A story about the research: Jafari, M., et al. “Bipartite network models to design combination therapies in acute myeloid leukaemia”.
Written by
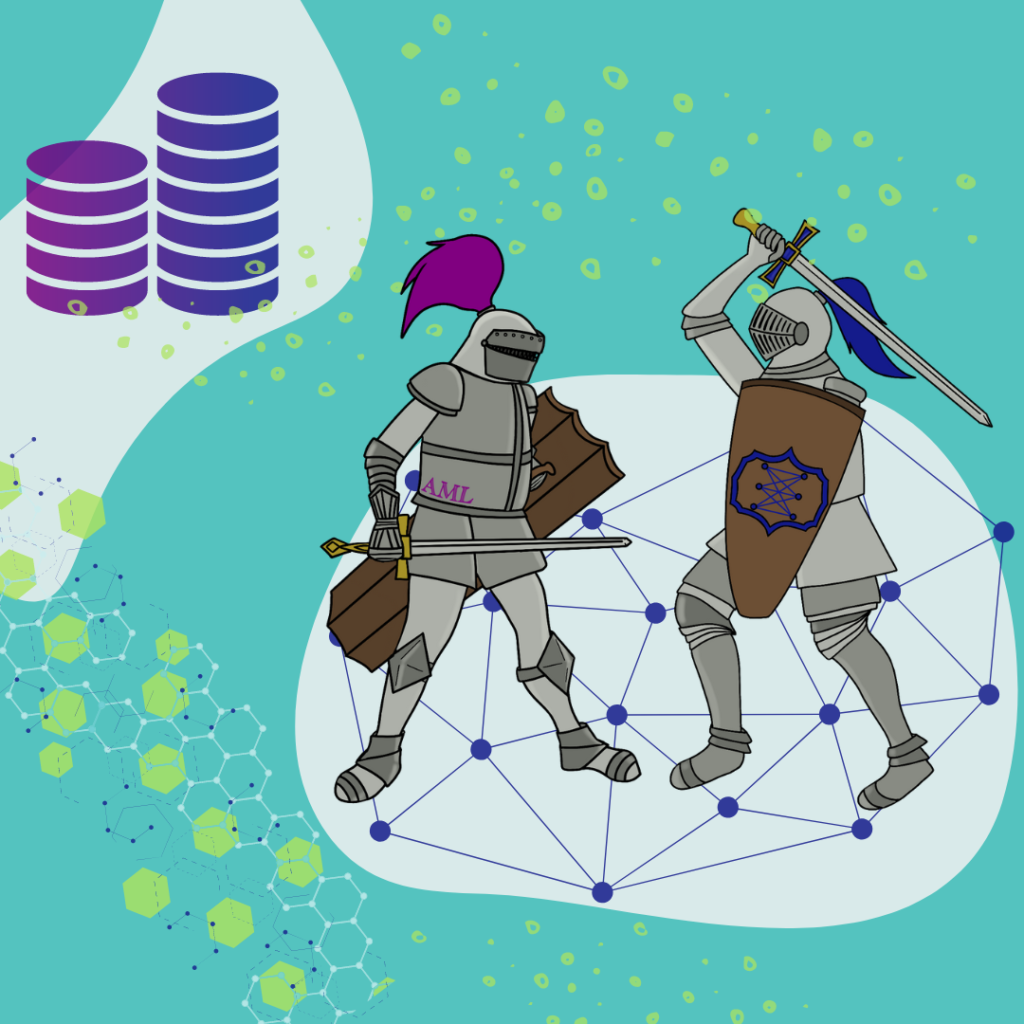
This blog post will answer these QUESTIONS:
- What is nominal data mining?
- What are the challenges in the design of combinatorial therapies in cancer?
- Did you know that nominal data mining can be used to plan combinatorial therapies for Acute Myeloid Leukaemia?
A prequel of the research
Imagine yourself sitting on a wooden platform during a sunny and humid day, watching a mediaeval knight’s tournament at Visegrád Castle in Hungary, when an idea of “bipartite networks” flashes across your head.
This performance was part of the interdisciplinary signalling workshop (ISW2017) in July 2017, which did not finish with sitting and watching. The conference participants were also becoming mediaeval knights, competing in combat skills in groups of ten with spears, swords, and other weapons. It was an opportunity for me not only to present my idea to other fellow scientist friends and colleagues, but also to have time to relax, have a few laughs together, and get enthusiastic about challenging training goals.
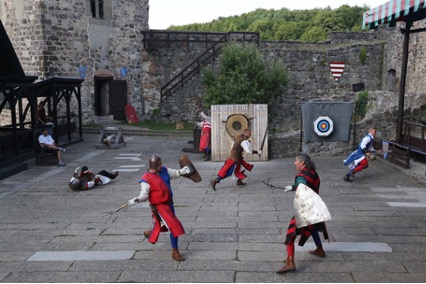
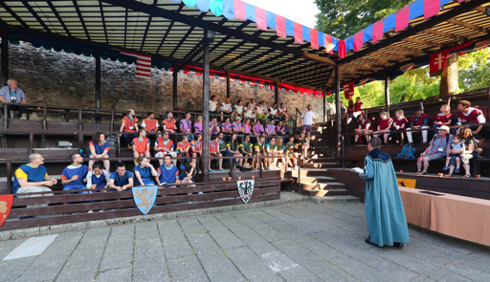
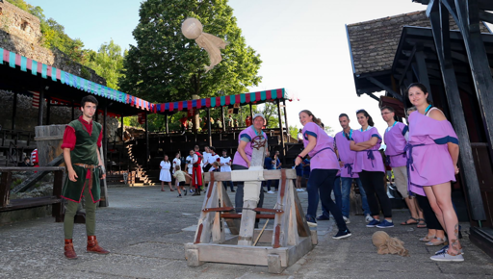
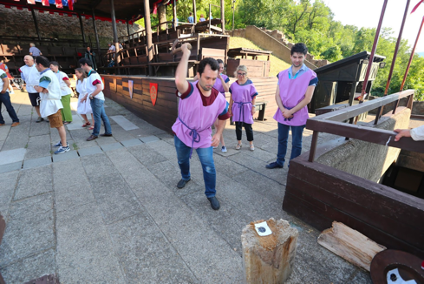
My first memory of conceiving the idea of ‘bipartite networks’ was here. I was following the instructions to build teams for competing in combat skills and at the same time my mind was adrift in lecture content. During the event, multiple invitees noted the increasing prevalence of extensive databases in precision medicine that contain diverse types of variables, such as nominal and ordinal variables (Types of variables in a nutshell). Consequently, there is a rising demand for specialised biological data mining techniques to explore more information. Meanwhile, I realised that we already built ten different teams from conference participants just by randomly choosing coloured mediaeval vests. “Look, here’s a dataset with two nominal variables (participants and coloured vests), which can be modelled as a bipartite network. Is this team-building exercise and person-to-colour assigning completely random? Is it possible to predict which team will win? And so on,” I was talking to myself.
This idea gradually developed until I joined the Institute for Molecular Medicine Finland (FIMM) at the University of Helsinki as a senior researcher in October 2018. I was surprised at being told upon arrival to prepare a grant proposal. However, I quickly realised that thanks to a collaboration with Caroline Heckman, I had access to datasets at FIMM with the exact attributes I required to implement my idea! I had access to the big datasets containing multi-level nominal variables based on patients! It was at this point that I felt it was the right time and the right place to focus on a cancer disease like acute myeloid leukaemia (AML), which has a poor prognosis and requires new treatment options and drug combinations. In AML, traditional chemotherapeutics have limited efficacy in patients over the age of 65 years, with a survival rate of less than 25% in a year following diagnosis, and less than 9% of those individuals surviving for 5 years.
Nice plots and what do they really mean
The primary goal of the study from the outset was to gather additional information from patients based on the treatment response of ex vivo (outside of the living body) samples to recommend their effective/potential drug combinations. The study design was based on the modify(verify)-measure-mine-model paradigm, which has been accompanied by prediction verification (Figure 1).
- Modify and Measure: The researchers at Oregon Health & Science University completed these two parts as we utilised the BeatAML dataset in this pilot study prior to working with the FIMM dataset.
- Mine and Model: After pre-processing, drug response datasets that contain a significant number of patients and drugs were used to construct bipartite networks. Preparations were made to recommend beneficial therapeutic combinations by clustering patients and drugs. Other supporting evidence for the validity of the predictions was assessed using prior knowledge and the available dataset of drug combinations (e.g., ALMANAC dataset).
- Verify: To further validate the predictive capability of our model in identifying effective drug combinations, we conducted an experimental confirmation on a specific subset of 45 drug combinations across three cell lines associated with acute myeloid leukaemia (AML), namely MOLM-16, OCI-AML3, and NOMO-1.
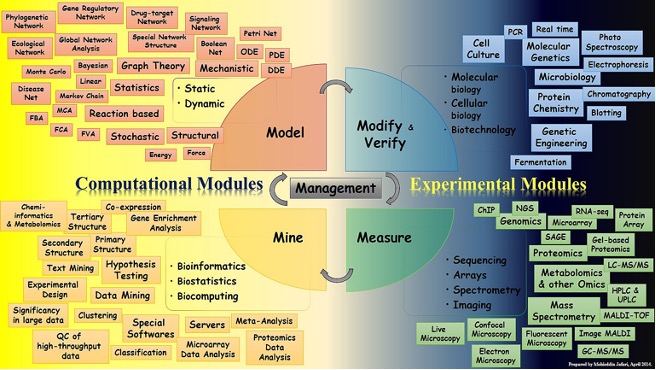
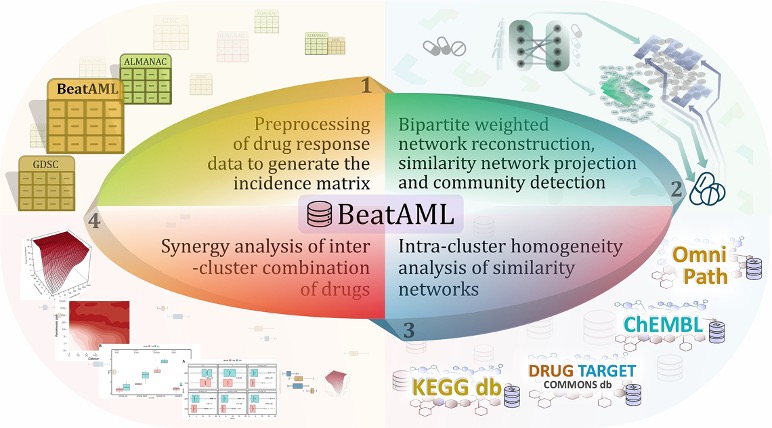
As a result, this can be considered a typical systems biology study since it includes thorough computational and experimental modules, consistent with a holistic view of systems biomedicine that incorporates interconnections between entities. The key to achieving acceptable and relevant results in such multidisciplinary studies is to use a common language and to communicate effectively among researchers across distinct disciplines. For effective research collaboration, it is essential that researchers from diverse backgrounds have a comprehensive understanding of the entire analysis process, as opposed to viewing it as a series of isolated steps or black boxes. Typically, this type of research begins with the identification of a problem in a biomedical topic, followed by the application of solutions from disciplines such as computer science, mathematics, and statistics. This approach promotes necessary interactions and ensures that researchers can contribute their expertise to the overall analysis. By sharing a certain level of information about the analysis procedure, collaboration can flourish and produce more significant results. Having people with an interdisciplinary background in a team to build effective communication has always shown to be a winning formula.
Of course, we also used this formula. We conducted an investigation to explore the potential of utilising common measurements for drug response, specifically AUC (Area Under the Curve) and IC50 (Half-Maximal Inhibitory Concentration) (Figure 2). These measurements are commonly employed by researchers to develop computational models. However, considering the limitations and challenges associated with AUC and IC50, as detailed in the article, we ultimately developed our model by selecting the median of cell viability as an alternative criterion. In the analysis of drug sensitivity on cell culture, the metric of cell viability refers to measuring the proportion of cells that remain alive after being exposed to a specific drug or compound. This metric is utilized to gauge the drug’s impact on the survival and proliferation of cells in the culture. The decision to use the median of cell viability was made to enhance the comparability of drug responses and address the issues identified with AUC and IC50. It is important to note that blindly adopting either AUC or IC50 measurements without a thorough understanding of their implications within our dataset would have led to significantly different outcomes. By critically evaluating their relevance and limitations, we were able to tailor our model to provide more accurate and meaningful results. Thus, by combining the expertise of researchers from various fields and employing a comprehensive understanding of the analysis process, we can overcome challenges and optimise the outcomes of our research.
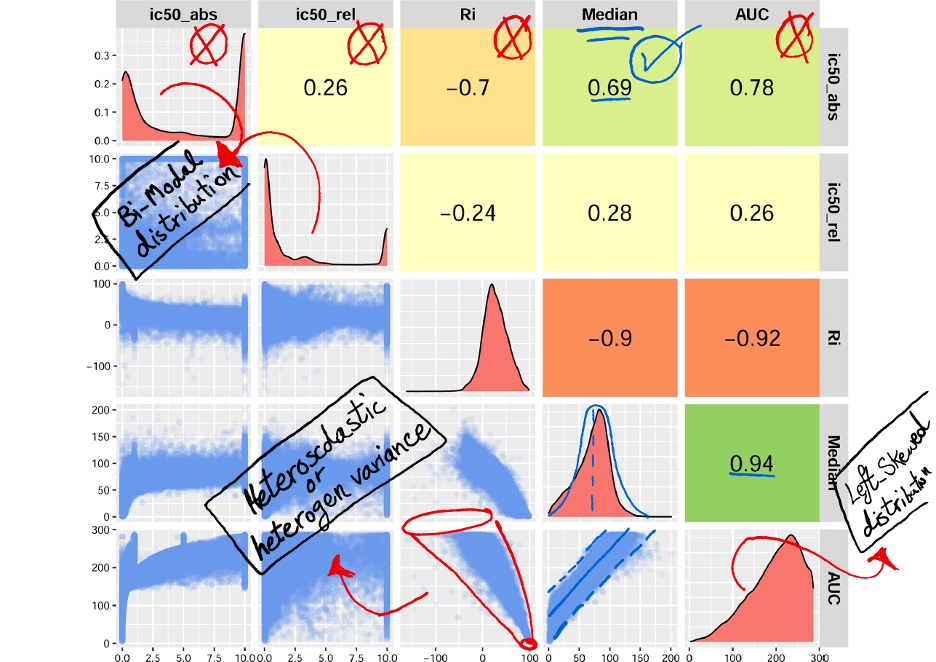
While achieving promising results on patient clustering and drugs, confirmed by independent evidence, the nature of the data drew my attention once more. I am referring to the nominal nature of the data that I used to build and analyse the model. Indeed, I was doing nominal data mining, and this method could be used to extract information from other datasets with at least two nominal variables with several levels. For instance, some of the similarity measures computed for drugs and for patients in this study are noteworthy and could be the subject of further research. Consider the following plots displayed in Figure 3, which show drug similarity based on prior molecular knowledge such as SMILES structure (Simplified Molecular Input Line Entry System) and target proteins. Notably, the similarity of drugs after being clustered into two distinct groups, as opposed to random grouping, is remarkably higher (Figure 3). This finding suggests that clustering drugs based on their response has the potential to create homogeneous clusters, aligning with prior molecular knowledge. Note that our findings are based on the drug sensitivity analysis of a single type of disease, i.e., AML, and these drugs are thought to be functionally similar (mostly kinase inhibitors).
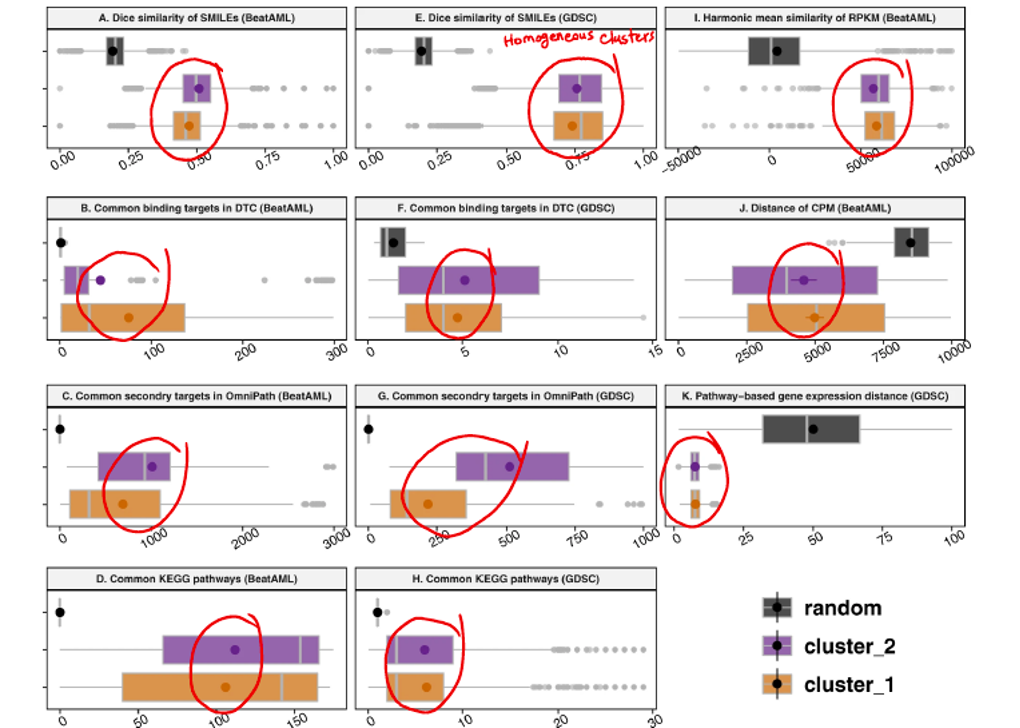
Instead of focusing on drug additivity, we calculated various synergy measures to assess the synergistic behaviour of the drugs. Drug synergy occurs when the combined effect of two or more drugs is greater than the sum of their individual effects, resulting in a more enhanced therapeutic outcome compared to what each drug can achieve independently. This phenomenon is desirable in medical research and treatment because it enables the use of lower doses of drugs while still achieving effective results. To evaluate their effectiveness, we divided the drug combinations into positive and negative groups based on whether they came from different clusters or the same cluster, respectively. We then analysed the synergy and sensitivity scores of these combinations, specifically considering metrics like highest single agent (HSA), zero-interaction potency (ZIP), Bliss, Loewe, combinational sensitivity score (CSS), and S synergy.
The results, depicted in Figure 4, clearly demonstrate that the positive group of drug combinations exhibited significantly higher levels of drug synergy compared to the negative group. This pattern held true across all synergy measures employed, highlighting the effectiveness of utilizing inter-cluster drug combinations. These findings also underscore the efficiency of our proposed network-based modelling approach in identifying drugs with similar effects on biological samples. Furthermore, our strategy of combining drugs from contrasting clusters showed a greater likelihood of achieving enhanced drug synergy and potency. These observations support the validity and potential benefits of our approach in optimizing drug combination therapies.
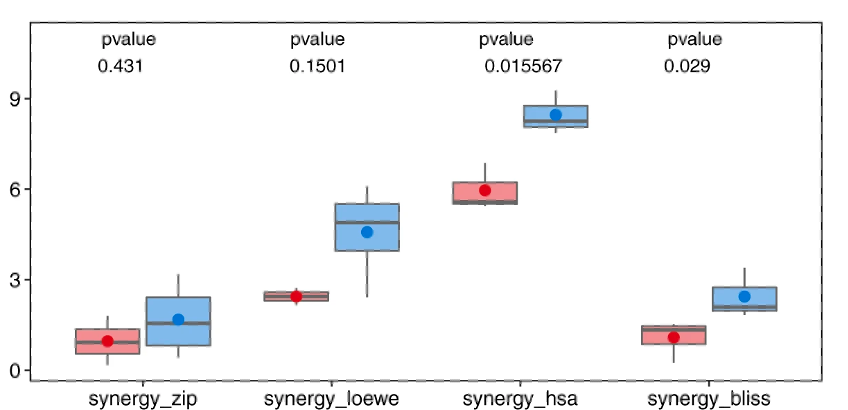

Furthermore, the strategy used in this study to propose drug combinations holds therapeutic promise, particularly in cases where the number of possible states for drug combinations is exponentially large. Taking patient heterogeneity into account, our approach would recommend various combinations that have the potential to be effective in the majority of patient samples. Experimentally validating the proposed drug combinations is not much of an operational hassle, especially in circumstances when automatic liquid handling systems can test a large number of drugs in a short period of time.
As a result, the idea that came to me during the mediaeval battle exercise at the conference has transformed into captivating scientific insights, leading to a publication in Nature Communication, which I am glad to share with readers.
Take-home messages
- Nominal data mining refers to the process of sorting through large data sets with multi-level nominal variables (at least two variables) to identify patterns and relationships between the levels through data analysis and network science.
- Due to the immense size of the drug combination space, it is essential to have a prior hypothesis or computational model that can help us prioritise and explore combinations to be tested efficiently.
- In this study, we demonstrated how nominal data mining based on network science can be used to design a combinatorial drug regimen for AML.
Links
Original thesis/article:
https://www.nature.com/articles/s41467-022-29793-5
Could be interesting to read:
- Malani, D. et al. Implementing a functional precision medicine tumor board for acute myeloid leukemia. Cancer Discovery, candisc.0410.2021, doi:10.1158/2159-8290.CD-21-0410 (2021).
- Tyner, J.W., Tognon, C.E., Bottomly, D. et al. Functional genomic landscape of acute myeloid leukaemia. Nature 562, 526–531 (2018).
- Palmieri R, Paterno G, De Bellis E, et al. Therapeutic Choice in Older Patients with Acute Myeloid Leukemia: A Matter of Fitness. Cancers (Basel). 2020;12(1):120. Published 2020 Jan 2. doi:10.3390/cancers12010120
- Palmer, A. C. & Sorger, P. K. Combination cancer therapy can confer benefit via patient-to-patient variability without drug additivity or synergy. Cell 171, 1678 (2017).
- Jafari, M., Chen, C., Mirzaie, M. & Tang, J. NIMAA: an R/CRAN package to accomplish NomInal data Mining AnAlysis. bioRxiv, 2022.2001.2013.475835, (2022).